Machine Learning Approaches to Classify Primary and Metastatic Cancers Using Tissue of Origin-Based DNA Methylation Profiles
Authors: Shakshi Sharma (University of Tartu, Estonia); Rajesh Sharma (University of Tartu, Estonia)
Early cancer diagnosis is critical for patients' early treatment and survival. Moreover, the cancer type and their primary origin are critical factors, as metastatic tumors account for up to 90% of cancer-related deaths.
Therefore, a clear distinction between the metastatic and primary cancers is crucial. Changes in DNA methylation are becoming more well-acknowledged as a factor in cancer prediction, particularly when it comes to the shift to metastasis. Thus, in this study, we utilized 24 cancer types and 9303 methylome samples from publicly available repositories such as The Cancer Genome Atlas (TCGA), and the Gene Expression Omnibus (GEO). After preprocessing and feature selection of the dataset, we visualize our high dimensional methylome dataset using dimensionality reduction techniques such as PCA and t-SNE where we reduce the dimensions to two.
The AI sector has shown promise in cancer classification throughout the last decade. Based on publicly accessible DNA methylation data, we used various machine learning techniques (a branch of AI); Naive Bayes (NB), Support Vector Machine (SVM), Random Forest (RF), and XGBoost (XGBoost) to identify cancer type based on their tissue of origin.
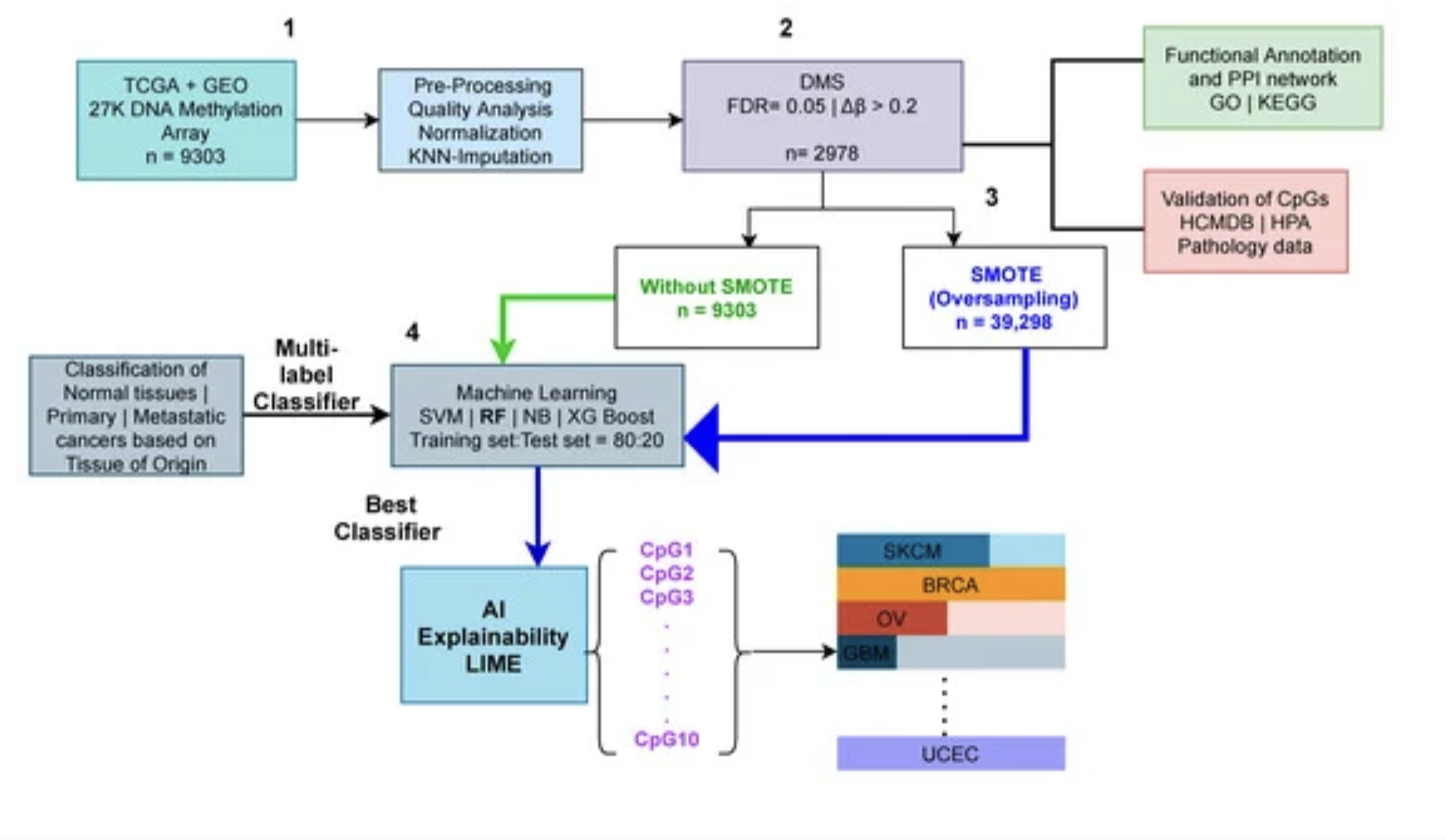
To train these machine learning models, we divided our dataset into 80:20 train and test sets. We also employed the Synthetic Minority Oversampling Technique (SMOTE) to achieve a balanced dataset because the number of methylome samples from each class was not equal. We also utilised the K-fold cross-validation technique on the train set to eliminate any potential bias. As a result, we ran the multi-label prediction tasks both with and without the SMOTE method. The findings of our trials show that the SMOTE strategy produces superior results than the non-SMOTE approach.
Finally, we report the results of the test set after the SMOTE technique for the Random Forest classifier. We employed five evaluation metrics; accuracy, precision, recall, f1 score, and aucroc score. Overall, our research found that predicting cancer subtypes based on the tissue of origin was 99 percent accurate. Specifically, RF outperforms all other classifiers.
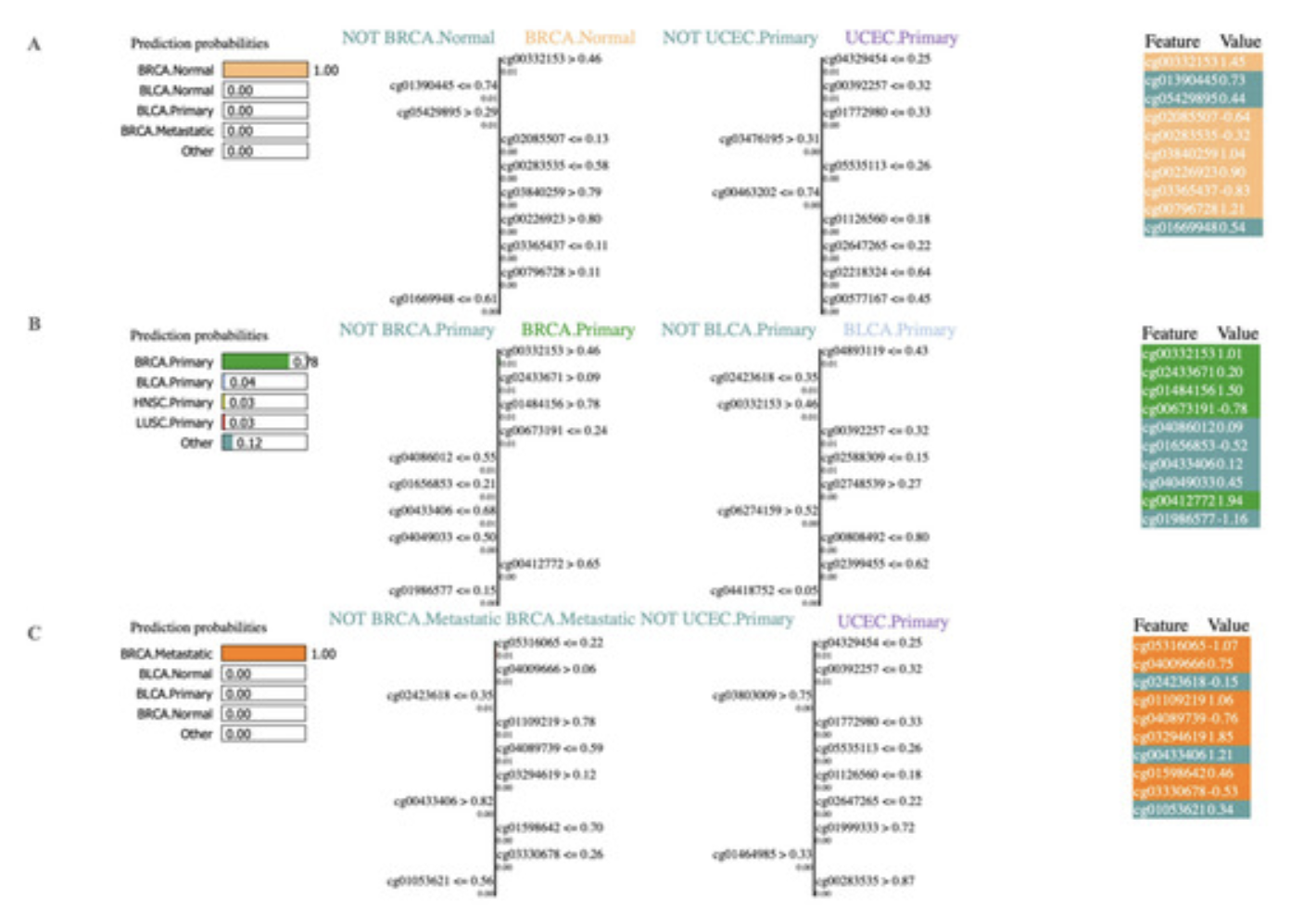
We did not stop our analysis here, next, we employed the AI explainability tool, Local Interpretable Model-agnostic Explanations (LIME), to classify cancer types using significant methylation biomarkers. In particular, we investigate the contributions of each feature using the LIME tool. The predicted classifier was one of the primary inputs to LIME, thus we used our highest-performing RF classifier in the LIME tool to get the contributions of the features.
This research not only aids in the automatic prediction of various types of cancers, but it also explains which features were most important in the prediction tasks.
This study has some limitations such as the fact that we did not have a sufficient balanced dataset for metastatic and normal samples to perform a robust classification though we used SMOTE to artificially balance the data by augmenting.
To summarize, we hope that the proposed model will be useful for cancer diagnosis, prognosis, and patient stratification. In the prediction of cancer types, a combination of AI-based techniques and methylome profiling data will be useful. Furthermore, the higher accuracy provided by this AI-based technology demonstrates its cost-effectiveness and applicability.