Charting content amplification through fake accounts and coordinated inauthentic behavior on Twitter
Authored by Michele Mazza (CNR-IIT)
Revised by Guglielmo Cola and Maurizio Tesconi (CNR-IIT)
Social media platforms are abused and misused, most commonly through fake accounts, giving rise to coordinated inauthentic behaviors (CIB). Even though efforts have been made to limit their exploitation, ready-to-use fake accounts can still be found for sale in a number of underground markets. In order to study the behavior of these accounts, we developed an innovative approach to detect and track accounts for sale. In the period between June 2019 and July 2021, we detected more than 60,000 fake accounts, which were tracked continuously for changes in their profile information and timeline. Afterward, we examined the 23,579 accounts that produced at least one tweet in 2020, identifying the main characteristics such as the most frequently used names and profile descriptions. Additionally, our analysis covered more than five million tweet interactions, including mentions, replies, retweets, as well as hashtags and URLs. The study of these patterns revealed behaviors indicating coordination, such as retweeting the same account. In this blog post, we describe a CIB that attempted to influence the political debate in the Buenos Aires Province.
Let us briefly show our innovative approach to detecting and tracking fake accounts. Twitter account merchants openly promote their products on their websites. A merchant we have studied, buyaccs.com, provides potential buyers with a list of sample accounts that are categorized based on their quality, which is based on how much an account seems a genuine and reputable source of information. In our system, the Accounts Collector module checks buyaccs.com for new account samples every two hours using web scraping. The Profile Updater module automatically updated the user object information for each collected account every six hours. Further, the Timeline Updater module downloaded the user's timeline every twelve hours.
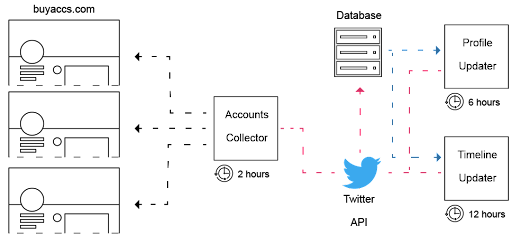
Figure 1: Schematic diagram of the automated system for detecting and tracking accounts for sale.
In light of the fact that among the most retweeted accounts, there were accounts related to the politics of the Buenos Aires Province, we searched the dataset for analogous retweeted accounts. As a result, 102 accounts were identified as being responsible for a specific coordinated inauthentic behavior. By retweeting 23 accounts related to Buenos Aires politics, these accounts served as amplifiers. Among these 23 retweeted accounts, 21 have been verified by Twitter.
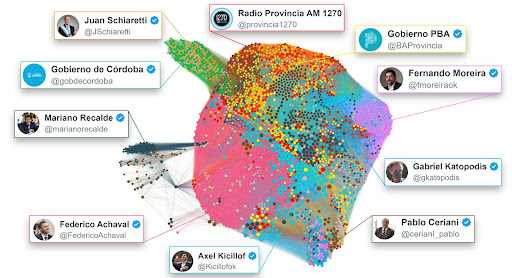
Figure 2: Graph describing how the contents from ten political accounts were spread by fake accounts through retweets. Each node represents a tweet, with size proportional to the number of received retweets. Nodes are linked only if they share at least one common retweeter. Color identifies the tweet's author. Homogeneous color regions suggest the presence of groups of fake accounts that were focused on retweeting only a specific politician among the ones considered in the graph.
During 2020, the accounts involved in this CIB produced 18,101 retweets: Figure 2 reports how the original tweets were amplified by the 102 fake accounts. Nodes in the graph correspond to tweets, with the size of each node being proportional to the number of retweets made by fake accounts. Two tweets are linked only if they share at least one retweeter (i.e., both tweets have been retweeted by a common fake account), and edges are weighted as a function of the number of retweeters they share. The color of the node highlights the author of the tweet. We colored only the tweets from the ten most retweeted accounts, since they represent 88.4% of all the tweets from this CIB.
In this way, the nature of the coordination can be better understood. There are large areas with a single color, suggesting that accounts such as @FedericoAchaval and @fmoreiraok were retweeted by accounts that did not retweet the other accounts. In contrast, tweets from @JSchiaretti and @gobdecordoba appear to be mixed and outside of the graph core, suggesting that these political accounts were often retweeted by similar fake accounts.
There is also an interesting observation to be made regarding the size of the nodes. There were significantly fewer tweets from @Kicillofok, the second most retweeted account, than from @FedericoAchaval, the most retweeted account. The reason is that @Kicillofok's tweets are represented by more prominent nodes, which indicates that they received more retweets. Also, the tweets from @marianorecalde appear to be spread throughout the graph in different areas, indicating that disjoint groups of accounts retweeted them.
It is evident from these different retweeting behaviors that content amplification is not achieved through simple retweeting activity, but rather through the intervention of groups of accounts that behave in a slightly different manner to accomplish the same overall goal. As a result, the CIB may be masked in some way, making it harder to detect. Lastly, we would like to emphasize that our data cannot prove that some of these accounts acquired the fake accounts directly. In this case, the only evidence is that fake accounts were exploited in order to influence the political discussion.
Please find more information on this study in our research paper entitled "Ready-to-(ab)use: From fake account trafficking to coordinated inauthentic behavior on Twitter."
The full dataset with the IDs of monitored fake accounts and their tweets is publicly available in the SoBigData++ catalogue at this link.