Dynamics of opinion polarization in social networks
For decades, researchers from different fields have been trying to understand how people form their opinions. With the rise of social media platforms, this quest has become even more significant, especially due to the emergence of some alarming and extreme phenomena, such as the polarization of opinions, online hating, etc.
Understanding how people form their opinions means designing a model that can reproduce the dynamics of opinion formation. To this aim, many models have been proposed, and only a few of them have been already validated in real social networks. One of the most popular is the Friedkin-Johnsen (FJ) model, whereby every person in a network forms his/her opinion depending on an average (weighted on his/her susceptibility attitude) between his/her initial opinion (prejudice) and the opinion of his/her neighbours. Thus, two factors play in it: the anchoring to the original prejudice and the susceptibility towards the neighbourhood’s opinion, which are weighted according to the social weights among nodes. Both mechanisms have sociological foundations: the anchoring reproduces the personal capacity or willingness of each person to depart from the prejudice; the susceptibility models the fact that people change their opinions to reduce the discomfort caused by disagreement with others.
A key question to test the validity of the Friedkin-Johnsen model is the following: does this model capture polarization? Is it possible to identify opinions that produce polarization?
In the literature, however, there are different variants of the model (see Table 1). In the most general form of the FJ model (which we denote as gFJ), each node iteratively updates its opinion balancing the anchoring to its personal prejudice and susceptibility toward the opinions of its neighbours. The balance between the two is controlled by a parameter, called susceptibility i, namely the vulnerability of node i to others’ opinion influence. The non-anchoring part takes into account the opinions of the neighbors and the opinion of the node itself at the step before, and these quantities are weighted by the strengths of its social ties (collected into the matrix W). The variational Friedkin-Johnsen (vFJ) is more restrictive, because when nodes update their opinion they do not take into account their current opinion. Apart from this fact, gFJ and vFJ are very similar. Finally, the restricted FJ (rFJ) is derived from vFJ but here there is no any way to control the anchoring part of the model, which is assumed to be assigned in the same way for all the nodes of the network.
Table 1
Each model is said to be polarizing when the polarization in the network increases during the opinion formation process. How is the polarization measured? The metrics of polarization can be classified into four classes. Metrics in the local class measure the total disagreement in the network as a whole, summing up the disagreement perceived by each node weighted by the social ties. The other classes comprise instead global measures and do not take into account the social relationships among nodes. The dispersion class considers polarization as the quadratic deviation of opinions at equilibrium from the average. The other two classes consider a slightly different semantic since they distinguish between “neutral” and “extreme” opinions. Since opinions span over [-1,1], the neutral opinion is the opinion 0, whereas the extreme ones are -1 and 1. Thus, the absolute class measures the polarization as the quadratic deviation from the neutrality. The total class measures the polarization as the absolute linear deviation from the neutrality. Besides polarization metrics, we also consider the concept of choice shift. A choice shift occurs when the sum (let’s say the average) of the initial opinion is different from that of the final opinion.
The research question we have tackled is whether, given an underlying social network, the different FJ versions lead to polarization for any of the classes described above. We have proved important results. First, all the variants of the model are locally depolarizing by design. Furthermore, the gFJ and vFJ are globally polarizing in general (if some non-restrictive conditions are met) and this proves that the Friedkin-Johnsen model is realistic, since it is able to reproduce polarization. Next, and even more important, we have identified how to obtain polarizing vectors, i.e. the initial opinions that lead to opinion polarization: we identified three different local maxima and the global maximum for the absolute class of polarization and a local and a global maximum for the total class of polarization. Finally, we have proved that the weaker version of the Friedkin-Johnsen model is also globally depolarizing and it does not induce a choice shift in the network. This means that this variant of the model is not realistic.
We have tested our results on two real-life social network graphs: the Karate Club graph and a Facebook subgraph. We needed to design a way to assign the susceptibility of each node (which does not come from the network itself) and, since these networks have both very few central nodes, we decided to correlate the susceptibility value according to their centrality, and to compare this configuration with one where all nodes have the same susceptibility. Thus, for the susceptibility, we evaluated three configurations: the individual susceptibility of a node is (i) proportional to its Pagerank centrality Pi, (ii) inversely proportional, and (iii) all susceptibilities are set to 0.8.
In Figure 1 we can see the shape of the polarizing vectors for the absolute class of polarization. Each node is represented by an arrow, whose starting point is the initial prejudice and ending point is the final opinion The three rows represent the three configurations of susceptibility: directly proportional to Pi, inversely proportional, and equal to 0.8. The first three columns are the local maxima, the fourth one is the global maximum.
We can see that the global solution is the one that features the maximum number of nodes with an extreme initial opinion (equal to 1): in this way, the nodes with more extreme opinions work synergistically to push the others’ opinions closer to theirs.
For what concerns the centrality, instead, we can see that when the susceptibility is set according to the centrality of nodes, the nodes with larger opinions are those more stubborn (so that they can influence more the neutral nodes toward their opinion). In the third row, where the susceptibility of all nodes is the same(panel C of Figure 1), the polarizing vectors that maximise polarization (and so that are able to influence more the opinions of nodes) are those in which the most central nodes have initial opinions extreme. This confirms that the PageRank centrality is able to capture the ability of nodes to convince the others, and thus it identifies the most influential nodes.
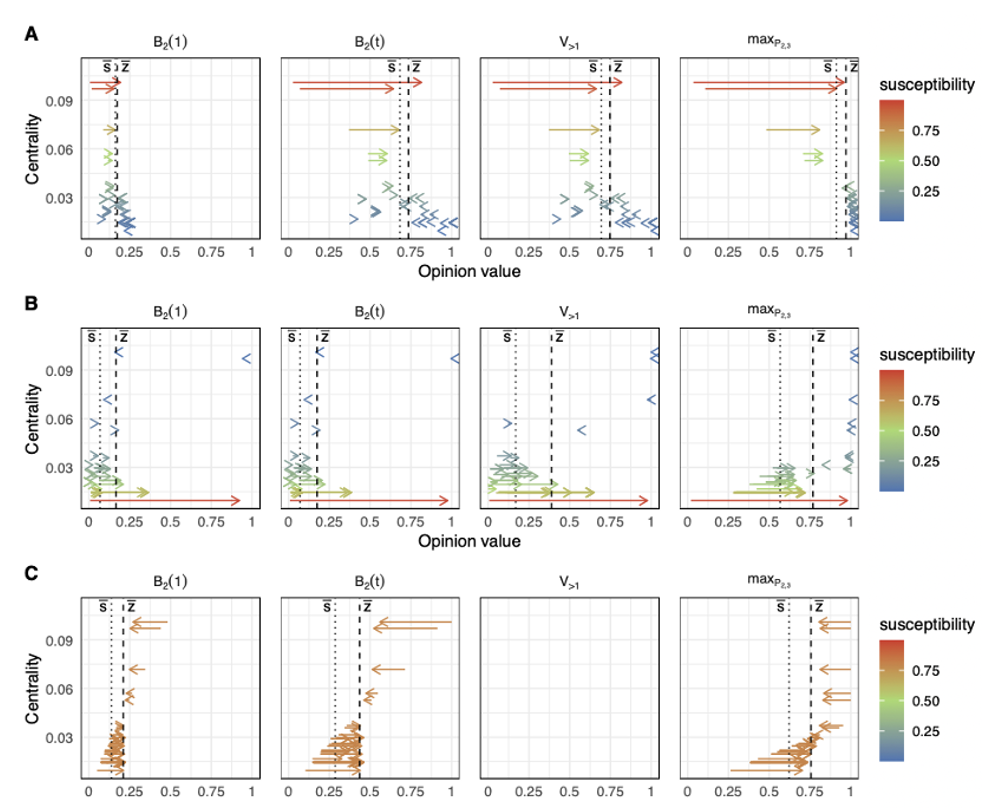
Figure 1: gFJ on the Karate network: polarizing opinions vs nodes centrality. Each arrow corresponds to one node in the Karate graph. An arrow starts at the initial prejudice and ends at the final opinion. The colour of the arrow corresponds to the susceptibility assigned to the node. (A) susc. ∝ Pi (B) susc. ∝ Pi−1 (C) susc. = 0.8. With the dotted and dashed line, we denote the average initial and final opinion, respectively.
In conclusion, in this work, we have done a comprehensive revision of all the variants of the FJ model and have proven that they are not equivalent. Our findings show that the simplified version of the model, despite being commonly used in the related literature, does not reproduce polarization, while under the original model proposed by Friedkin and Johnsen, polarization can emerge. This is fundamental for an opinion dynamic model to be realistic. Furthermore, we provide the conditions under which polarization happens as well as discuss how to find the initial opinions leading to polarization. We have finally seen that both susceptibility and centrality of nodes play a role in polarization.
Author: Elisabetta Biondi
Exploratory: Societal Debates
Sustainable Goals: 13. Promote peaceful and inclusive societies for sustainable development, provide access to justice for all and build effective, accountable and inclusive institutions at all levels:
- target T.1 Significantly reduce all forms of violence and related death rates everywhere: we have studied how polarization occurs over social graphs