Gene expression Partial Correlation in chromosome 4 COPD patients
Author: Michele Gentili.
Chronic obstructive pulmonary disease (COPD) is a complex disease influenced by environmental exposures (most notably, cigarette smoking) and genetic factors. Genome-wide association studies have identified thousands of genomic regions associated with complex diseases. The chromosome 4q region harbors multiple genetic risk loci for chronic obstructive pulmonary disease (COPD). To determine whether genes in this region are part of a gene expression network, we studied lung tissue RNA-Seq from COPD cases and controls.
It is likely that the effects of genetic variants in complex diseases cannot be captured by a single type of omics data. However, including different biological data sources may facilitate the removal of indirect effects, allowing the identification of correlations that would not be detected otherwise.
We leveraged protein-protein interaction information to build a partial correlation network, controlling for all of the genes in the genome while assessing for the correlation between pairs of genes.
Gene Specific Partial Correlation
Due to the computational complexity of the problem, we cannot compute the partial correlation score controlling for all of the genes at the same time. Indeed, the ratio between the number of regressors (p), i.e., the number of genes in the genome ~20k, and the number of observations (n), i.e., the number of samples in the study (usually ~1k), is well above 1 (p>>n), which makes the calculation of the inverse matrix indeterminate.
In this work we introduce a gene-specific regularization factor when computing the Partial Correlation score to make the indeterminate regression feasible. We decided to slightly modify the computation of the sparse partial correlation matrix (Figure 1). Controlling for other genes’ expression we take into consideration additional biological sources, such as the PPI or co-occurrence in biological pathways, to prioritize which genes would have a greater weight, i.e., using a specific λ_(i,k) when computing partial correlations for gene i (gi) and controlling for gene k (gk). The higher the score in λ_(i,k) (for instance genes far away in the PPI) the less gk will be considered to regress gi expression.
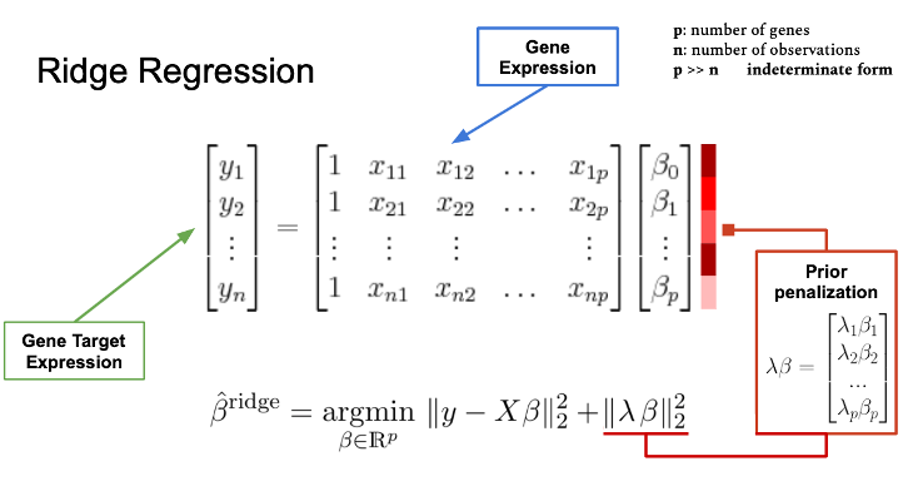
Figure 1 Graphical explanation of the Gene Specific Ridge Regression. The main novelty is that λ, rather than being a scalar, is a vector. Each element λ_i penalizes the coefficient β_i individually. In this way we provide more importance to “biologically” close genes.
We found significant partial correlations in gene expression between genes across chromosome 4q extending beyond the genomic distance covered by a single topological associated domain. Of interest, similar long-range partial correlations were found in other genomic regions, which suggests that long-range gene regulatory mechanisms may be widespread in the genome. Although we did not find a significant overall difference in network edges between COPD cases and controls within the chromosome 4q region, long-range gene regulatory effects were implicated.
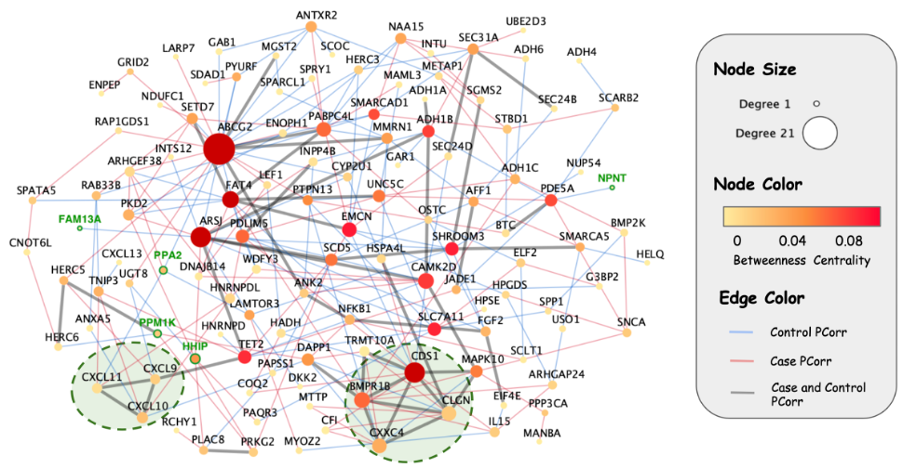
Figure 2 Partial Correlation Network, chromosome 4q region. Blue edges represent pairs of genes that are partially correlated only in the control subjects, red edges are partially correlated only in COPD subjects, and gray edges are statistically significant in both populations. CCG genes have a green ring around them. The two dashed circles identify two cliques in the network.