Investigating Psycho-linguistic Patterns to Debunk Misinformation
From a TNA experience.
Author: Shakshi Sharma, Host Organization: University of Sheffield, UK. Mu University: University of Tartu, Estonia.
Online social media platforms like Twitter specifically allow for the open exchange of ideas but have not been able to prohibit the spread of misinformation. Studies have demonstrated that on these platforms, false information spreads six times as rapidly as actual news, causing society to worry, anxiety, and financial loss [1]. To stop the misinformation spread, there are multiple techniques followed by researchers. This could include but is not limited to content-based [2, 3], context-based [4, 5], and network-based approaches [6, 7]. To fully understand the phenomenon of misinformation and comprehend the continually growing nature of social media, it is important to continue research in this field.
A comprehensive approach must be developed to combat the rampant misinformation spread, and one effective strategy is to examine the psycho-linguistic behavior of those disseminating misinformation (misinformation imposters) and those actively working to debunk it (active citizens). By developing a greater understanding of the motives, patterns, and strategies used by these groups, it would be feasible to create targeted interventions that can successfully reduce the spread of misinformation.
Also, platforms can use sophisticated algorithms and machine learning models, for instance, to identify and flag possible misinformation imposters based on language patterns, dubious behaviors, and content analysis. Additionally, teaching the public about the psychological strategies used by misinformation imposters might improve critical thinking abilities, helping people to more accurately assess the reliability of the content they come across.
To achieve this goal, I visited the laboratory of Assoc. Prof. Nikolaos Aletras at the University of Sheffield, UK. My stay there provided me with an opportunity to connect with a variety of individuals who each had a certain area of expertise and to have good conversations with them. I was pleasantly delighted to see that every team member was really kind and eager to assist with any issues. Apart from the frequent in-lab meetings with Prof. Aletras and his students, we also went out for lunch a few times during my stay and received various suggestions to eat and roam around the city.
We accomplished excellent progress during my visit as we worked quickly to address the numerous aspects of the research problem. First and foremost, we successfully gathered the required dataset, ensuring that we had reliable and complete data to work with.
We then established pertinent research questions that enabled us to meet the main goals of our study. We jumped right into the analysis stage after gathering the information and developing clear research questions. We started identifying important insights and patterns in the obtained data via careful inspection and investigation, which would later help us to draw conclusions from our research.
Apart from research, these profound connections among researchers that were established during the visit have an ongoing impact. It fostered trust and camaraderie, fostering continuous cooperation and potential future projects. A network of people with similar interests and a common dedication to increasing knowledge in the area was formed as a result of the interchange of ideas, sharing of knowledge, and support from one another. This atmosphere was fostered beyond the immediate research aims.
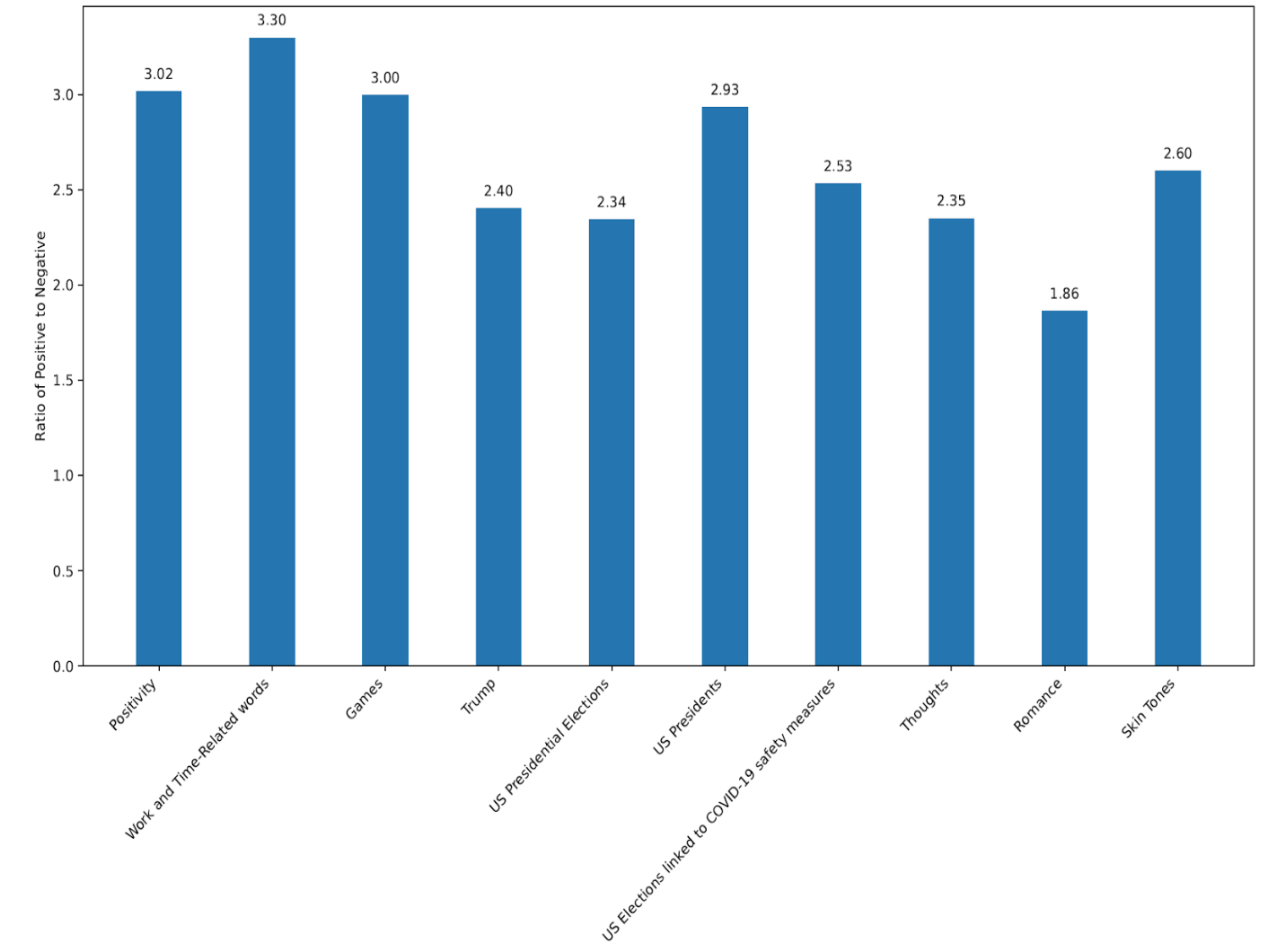
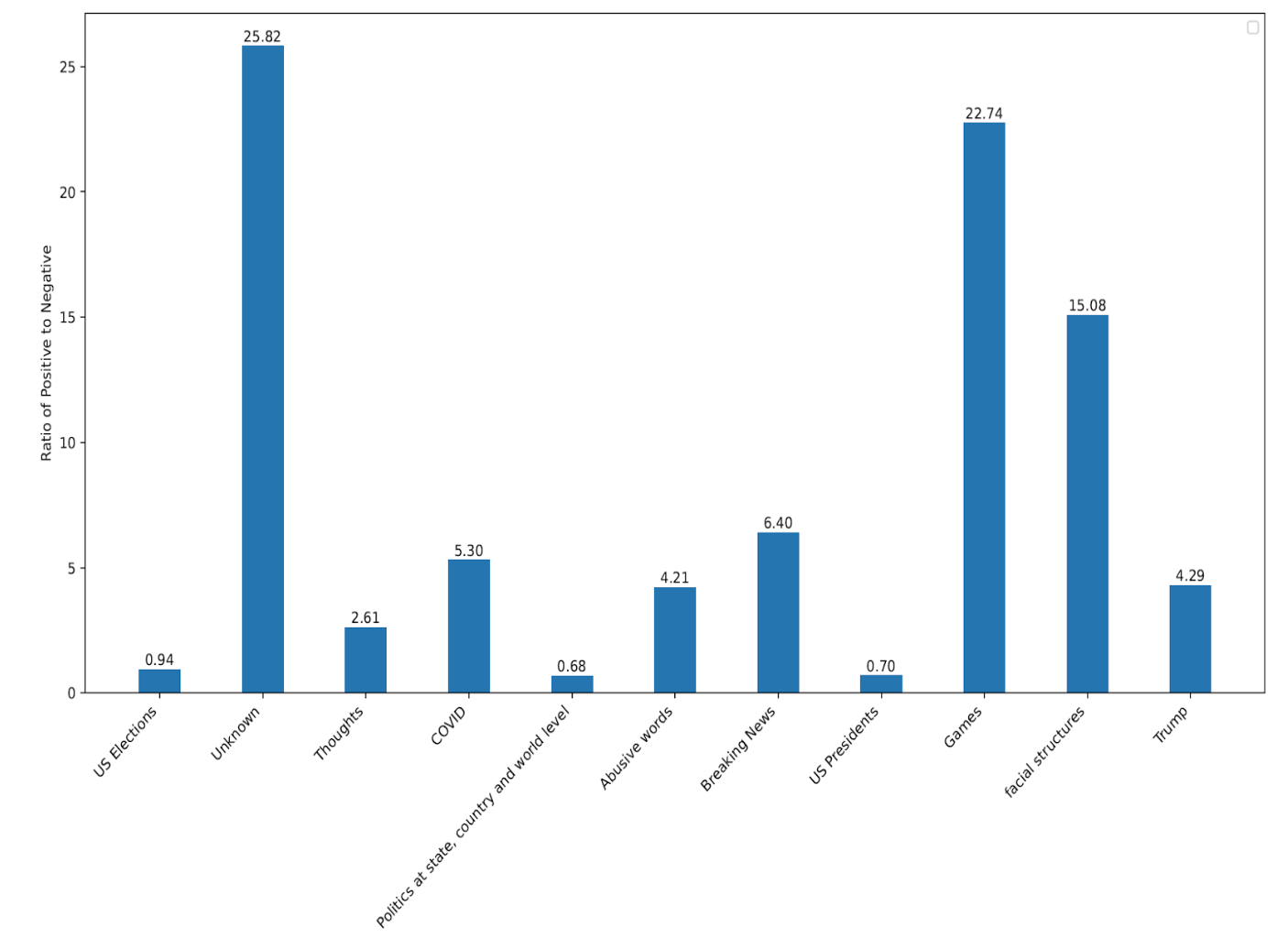
References:
[1] Sharma, Shakshi, and Rajesh Sharma. "Identifying possible rumor spreaders on twitter: A weak supervised learning approach." 2021 International Joint Conference on Neural Networks (IJCNN). IEEE, 2021.
[2] Jin, Zhiwei, et al. "Multimodal fusion with recurrent neural networks for rumor detection on microblogs." Proceedings of the 25th ACM international conference on Multimedia. 2017.
[3] Karimi, Hamid, and Jiliang Tang. "Learning hierarchical discourse-level structure for fake news detection." arXiv preprint arXiv:1903.07389 (2019).
[4] Wu, Kun, Xu Yuan, and Yue Ning. "Incorporating relational knowledge in explainable fake news detection." Advances in Knowledge Discovery and Data Mining: 25th Pacific-Asia Conference, PAKDD 2021, Virtual Event, May 11–14, 2021, Proceedings, Part III. Cham: Springer International Publishing, 2021.
[5] Hu, Linmei, et al. "Compare to the knowledge: Graph neural fake news detection with external knowledge." Proceedings of the 59th Annual Meeting of the Association for Computational Linguistics and the 11th International Joint Conference on Natural Language Processing (Volume 1: Long Papers). 2021.
[6] Gorrell, Genevieve, et al. "SemEval-2019 Task 7: RumourEval 2019: Determining Rumour Veracity and Support for Rumours." Proceedings of the 13th International Workshop on Semantic Evaluation: NAACL HLT 2019. Association for Computational Linguistics, 2019.
[7] Liu, Yang, and Yi-Fang Wu. "Early detection of fake news on social media through propagation path classification with recurrent and convolutional networks." Proceedings of the AAAI conference on artificial intelligence. Vol. 32. No. 1. 2018.