(Mis)leading the COVID-19 vaccination discourse on Twitter
An exploratory study of infodemic around the pandemic
Authors: Shakshi Sharma, Rajesh Sharma (University of Tartu, Estonia)
The ongoing discourse in social media has amplified the fears, uncertainties, and doubts (FUD) surrounding COVID-19 and the currently available vaccines, leading to an infodemic (a portmanteau of information and epidemic, referring to the spread of potentially accurate and inaccurate information about a disease spreading like an epidemic). In addition, vaccine hesitancy has been reported not only in several low and middle-income countries (LMIC) but also in the US and Russia, which were some of the countries at the forefront of COVID-19 vaccine research. The average vaccine acceptance rate in LMICs was reported to be 80.3%, compared to 64.6% in the United States and 30.4% in Russia.
In this work, we wanted to identify false information on social media in order to battle misconceptions about COVID-19. Specifically, we collected a corpus of COVID-19 vaccination-related tweets (over 200,000) over the course of seven months (September 2020 - March 2021). We curated a representative selection of 114,635 tweets relating to Covid-19 vaccination from an initial set of over 200,000 tweets, as well as two mutually exclusive subsets of 1246 and 1000 tweets manually classified in terms of whether they are Misleading or Non-Misleading. We used a Transfer Learning technique to categorize tweets as Misleading or Non-Misleading using a pre-trained Transformer-based XLNet model and manually validated with a random selection. We performed 2 step process:
Exploratory analysis: In the first step we analyze the dataset across three dimensions: (i) Language Exploration utilizing syntactic structure and the principal themes involved in both Misleading and Non-Misleading tweets, (ii) Opinion Study leverage the sentiments and emotions of both types of tweets, (iii) Effect of Visibility involves analyzing meta-data of the tweets. These dimensions provide us insights to distinguish Non-Misleading from Misleading tweets.
Classification & explainability: Next, we used these descriptive features as an input to the machine learning models in order to validate that these descriptive features are indeed significant in segregating the misleading and non-misleading features. Results show that the aforementioned analysis aided the identification of potential features which could be explicitly leveraged to classify tweets to determine whether they are Misleading or not. This explicit approach was compared against a black-box model (XLNet), and the mutual consistency of these approaches, aided with the explainability dimension of our approach, reinforces the credibility of the classifiers . The efficacy, as well as marginal contributions of a subset of features, were explored empirically for further validation.
We used this to compare and contrast the properties of misleading tweets in the corpus with non-misleading tweets. This exploratory research allowed us to create features (such as feelings, hashtags, nouns, and pronouns) that can then be used to categorize tweets as (Non-)Misleading using multiple ML models in a clear and understandable way. Specifically, various machine learning models with up to 90% accuracy are used for prediction, and the value of each feature is explained using the SHAP Explainable AI (XAI) tool.
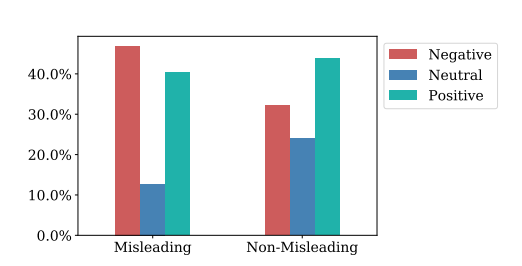
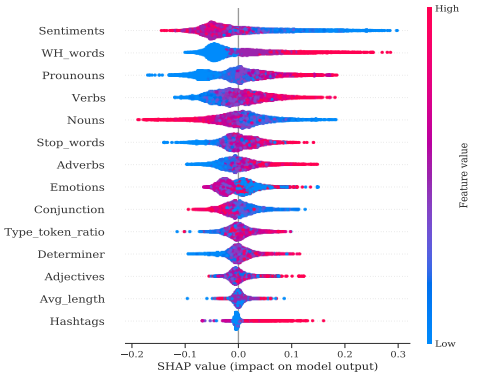
The complete work is available at this link