Quantification, Gelato, and Leaning Towers
Over the past two months, I have been conducting research at the Istituto di Scienza e Tecnologie dell'Informazione of the Consiglio Nazionale delle Ricerche (ISTI-CNR) in Pisa, Italy. During this time, my research focused on quantification learning, specifically on developing methods using deep learning. The SoBigData Transnational Access (TNA) program provided an exceptional opportunity to collaborate with some of the most renowned experts in the field of quantification and, without a doubt, I encourage other researchers to take advantage of TNA opportunities like this one.
My two months at ISTI-CNR were both enriching and productive. For those unfamiliar with the subject, Quantification Learning, also known as prevalence estimation, is a supervised machine learning method that consists of developing a model that is able to predict the prevalence of each class within a sample. Unlike classification, which focuses on predicting the class of individual observations, many real-world applications are more concerned with estimating the overall prevalence of different classes in a sample. Let me give you a few
examples of its use: for instance, rather than identifying the sentiment of each individual product review, the goal might be to estimate the percentage of positive, neutral, and negative reviews. Similarly, in environmental studies, the aim could be to determine the proportion of different plankton species in a water sample.
Traditional quantification methods often rely on predefined representations and loss functions, which can limit their effectiveness. Our research aimed to challenge these conventions. We explored how deep learning could be harnessed to create optimal representations tailored specifically for quantification tasks. We had studied the behavior of components such as the loss function, the feature extraction, how the samples are representated, etc, trying to find a combinarion that enhance performance in quantification. Instead of relying on standard methods, we leveraged deep learning to develop representations without being bound by traditional approaches. This innovative strategy aimed to uncover new ways to improve the accuracy and robustness of quantification models.
As a result of this research, we found that while the traditional approach yields good results, some of the methods we developed were more effective at capturing the underlying structure of the data. This led to more accurate prevalence estimates and allowed for greater generalization across different quantification tasks.
Beyond the research, the TNA program allowed me to spend two months living in Pisa, a small city full of charm where I always felt welcomed, thanks in part to the warmth and the friendliness of my colleagues at the CNR.
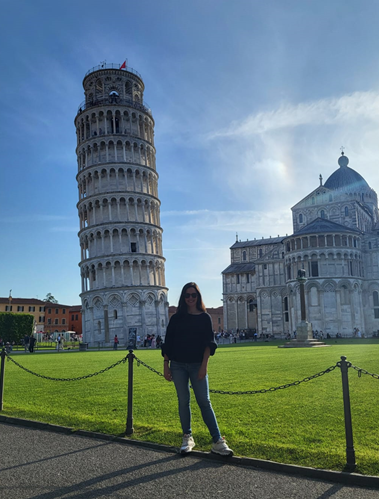
During my stay, I coincidentally got to experience Pisa's patronal festivities and I had the chance to experience the Luminara (as shown in the photo). Apart from the Torre Pendente, Piazza dei Miracoli and the Arno riverside, I also took the opportunity to explore the stunning Tuscany region, which fascinated me with its beautiful seaside as well as its rolling hills and countryside. I visited remarkable cities such as Florence, Lucca, Siena, San Gimignano, Volterra, Marina di Pisa, and many others, including the incredible island of Elba. However, there were still so many more villages left to explore.
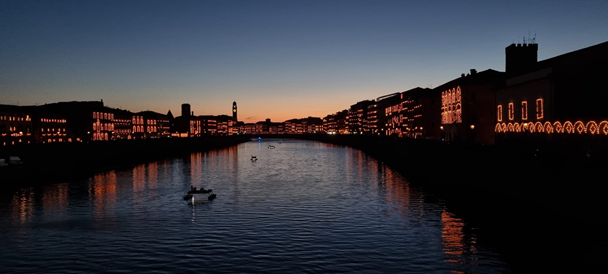
The delicious Italian food, pleasant weather, warm people, and beautiful landscapes made my stay in Pisa a truly memorable experience.