Charging Data Records are acknowledged as a standard tool for studying human mobility, infrastructure usage, and traffic behavior. We name such datasets as CdRs to distinguish them from the traditional Call Detail Records (CDRs), describing call and SMS cellular communication only. CdRs describe time-stamped and geo-referenced event types (i.e., data, calls, SMS) generated by each mobile device interacting with operator networks.
Social networks enable us to stay in contact with people all over the world, and to read news related to events that occur over the globe in almost real-time. While this has many positive impacts, such as allowing the report of adverse events. Unfortunately, such speed of diffusion of information over social networks has also important negative implications. In many cases malicious users may try to take advantage of the structure of social networks to spread misinformation.
An exploratory study of infodemic around the pandemic
Authors: Shakshi Sharma, Rajesh Sharma (University of Tartu, Estonia)
The ongoing discourse in social media has amplified the fears, uncertainties, and doubts (FUD) surrounding COVID-19 and the currently available vaccines, leading to an infodemic (a portmanteau of information and epidemic, referring to the spread of potentially accurate and inaccurate information about a disease spreading like an epidemic).
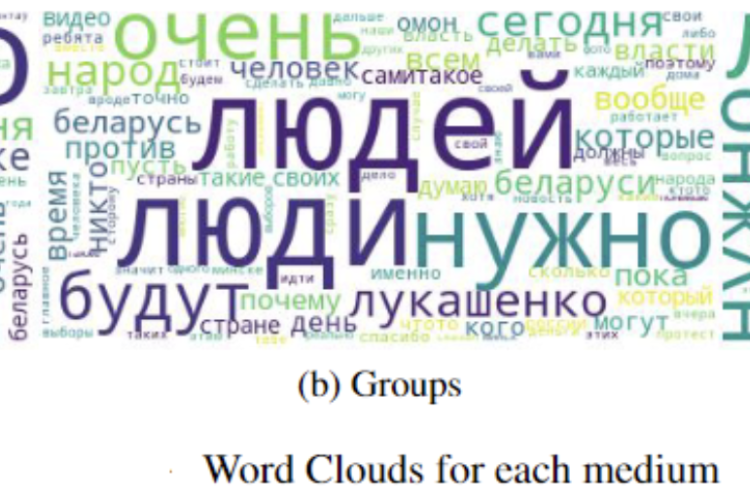
Authors: Ivan Slobojhan; Rajesh Sharma (University of Tartu)
More and more anti-government protests have occurred in the world in recent years. A common denominator for such protests is that they all rely on social media.
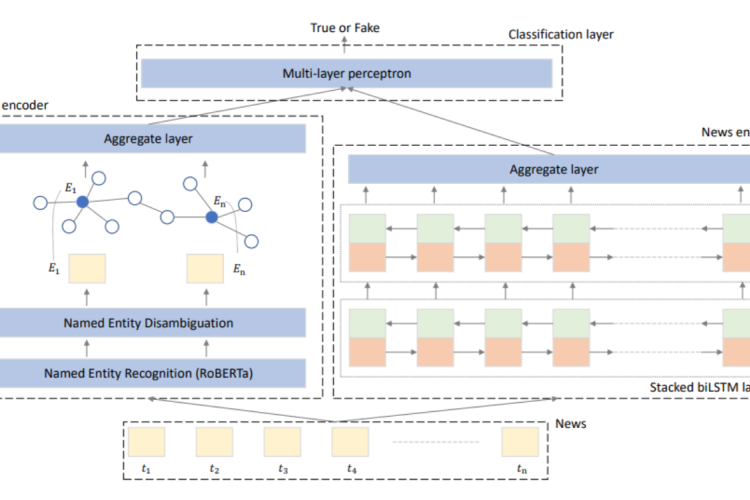
Authors: Shakshi Sharma (University of Tartu, Estonia); Rajesh Sharma (University of Tartu, Estonia)
Fake News on social media platforms has received a lot of attention in recent years, notably for incidents relating to politics (the 2016 US Presidential election) and healthcare (the COVID-19 infodemic, to name a few). Several approaches for identifying fake news have been presented in the literature. The methodologies range from network analysis techniques to Natural Language Processing (NLP) and the use of Graph Neural Networks (GNNs).
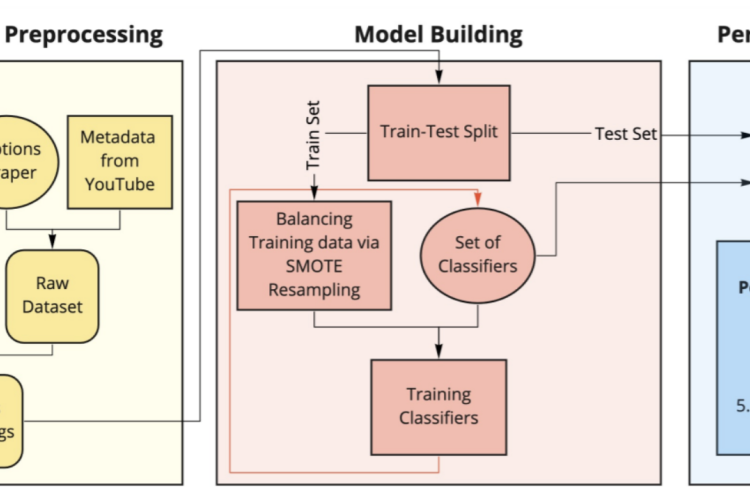
Authors: Shakshi Sharma (University of Tartu, Estonia) Rajesh Sharma (University of Tartu, Estonia)
In present times, Online Social Media (OSM) platforms such as Facebook, YouTube, and Twitter, have been used by billions of individuals for establishing a narrative, conducting propaganda, and disseminating misinformation.
The recommender systems employed by digital media platforms typically showcase content that is similar to the content a user has already consumed. When that content is biased, this runs the risk of fuelling radicalization. One option to mitigate the radicalization risks posed by digital media platforms is to modify their recommendations.
On November 18th, a special session dedicated to the SoBigData Research Infrastructure was held as part of the second edition of the International Forum on Digital and Democracy, a two-day conference which has the purpose of convening academics, researchers and policymakers to discuss the relationship between digital technologies and democratic institutions and to debate and exchange information, ideas and good practices about Digitalization and Democracy.